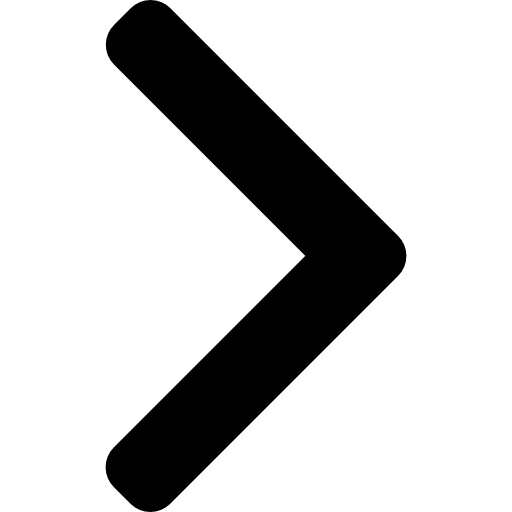
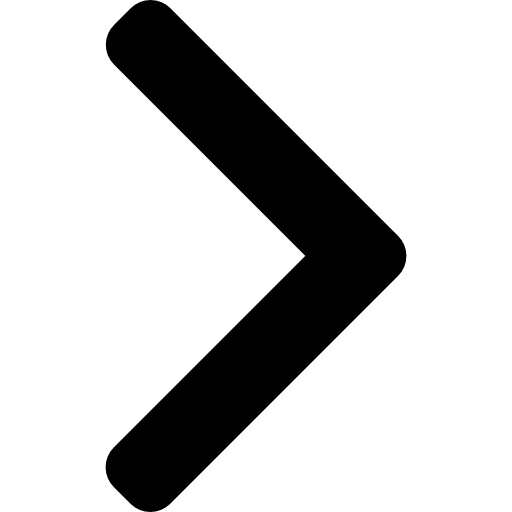
Deep Learning revolutionizes how we view agriculture in today's ever-advancing technology. By applying sophisticated deep learning algorithms to agricultural data, Deep Learning technologies can help farmers get the most out of their crops and increase production while improving the yield quality. By leveraging the power of artificial intelligence (AI), Deep Learning can bring about new techniques and strategies to tackle the challenges of modern agriculture.
Deep learning technologies can help farmers take their agricultural production to the next level. Farmers can create more efficient irrigation systems through Deep Learning, identify and rectify soil nutrient deficiencies, and even predict weather patterns to adjust their planting strategies. By utilizing Artificial Intelligence in agriculture with Deepcrops and solutions, farmers can increase their yields, improve the quality of their crops, and reduce their production costs.
Deep Learning, a branch of AI, is used to develop various neural networks to improve agricultural production. Neural networks are computing systems modeled after the human brain and can learn from large amounts of data to recognize environmental patterns. Deep Learning algorithms detect crop diseases, predict crop yields, and optimize irrigation scheduling.
Deep Learning algorithms can identify patterns in data that would otherwise remain hidden quickly. For example, an algorithm can detect a crop disease by scanning a field of crops for visual cues and then using Deep Learning to recognize patterns in the data that may indicate an infection. Additionally, Deep Learning predicts harvest yields by analyzing soil composition, weather conditions, and crop health data. Artificial Intelligence in agriculture allows farmers to plan how much food they can expect to harvest, ensuring they have enough to meet the needs of their customers.
Using Artificial Intelligence in agriculture like Deep Learning, farmers can also optimize their irrigation scheduling. Deep Learning algorithms can determine the best times to irrigate the fields by analyzing weather conditions, soil moisture, and crop health. It ensures that the crops are getting the right amount of water at the right time, improving the health of the plants and leading to higher yields.
Artificial intelligence (AI) in agriculture has proven to be an invaluable tool for modern farmers. Deep Learning has revolutionized the agricultural industry, allowing farmers to make more informed decisions based on data-driven insights. By utilizing Deep Learning algorithms, farmers can improve their yields, reduce the risk of crop diseases, and optimize their irrigation scheduling. It will continue to be a vital agricultural industry for years. Here are a few of the most commonly used deep learning neural networks:
Artificial Neural Networks: are one of the most common Deep learning algorithms applied to agriculture. ANNs are similar to biological neural networks in the human brain. ANNs identify patterns in data, such as images of healthy crops versus unhealthy crops, to identify crop diseases.
Convolutional neural networks: Convolutional neural networks are another standard algorithm used in agriculture. CNNs are commonly used to classify images but can also be used to predict crop yields based on weather data.
Recurrent neural networks: are used for many applications of AI in agriculture sector, such as predicting crop disease or optimizing fertilization applications based on soil composition data.
Natural language processing: Natural language processing is another standard tool of AI in agriculture sector for analyzing agricultural data. Computers can generate insightful agricultural insights by parsing and analyzing large amounts of data, including weather patterns and crop health.
Contextual inference: Contextual inference is another standard Deep learning algorithm applied to agriculture. CI makes predictions based on data from many different sources and is a helpful tool for crop variety selection.
Graphical processing units: Graphical processing units are a relatively new tool in Deep Learning but are quickly gaining traction in the agricultural industry. GPUs accelerate the rate at which ANNs and other deep-learning algorithms train and generate insights.
IoT, robotics, and processing technologies are very helpful to farmers. It provides high-quality images, and AI-based drone technology is very beneficial for farming because it makes monitoring, scanning, and analyzing crops simpler. Deep Learning technology helps to track the development of the crops. AI in farming can help farmers choose whether the crops are ready for harvest. The uses of Deep Learning and Artificial Intelligence in agriculture are:
As AI in agriculture sector advances and technology transforms the industry, the end of Deep Learning is bright. With AI and Deep Learning advancements, farmers can leverage new solutions to tackle age-old agricultural challenges. With Deep Learning in agriculture, farmers will be able to accurately identify pests and diseases in crops, optimize fertilizer use, and manage soil health. Deep Learning in agriculture can help improve agricultural production while also reducing costs. The future of Deep Learning is genuinely limitless.
Deep Learning can transform agricultural production, making it more efficient and productive. As more and more data is collected, Deep Learning generates even more solutions. Farmers will be able to more accurately pinpoint issues in their crops' soil, nutrients, and health. It can help improve production while also saving farmers money.
Modern agriculture uses cutting-edge technologies to boost output, cut costs, and boost revenue, including machine learning, Deep Learning, IoT, and robotics. Farmers primarily use Deep learning technologies to improve crop management and various applications of agriculture because rain, cyclones, floods, climatic changes, weeds, pests, and a lack of labor are some factors that affect crop growth in the agriculture field. Deep Learning will, in the future, have a notable impact on the agricultural sector.
You can subscribe to our blog to learn more about AI and Deep Learning.